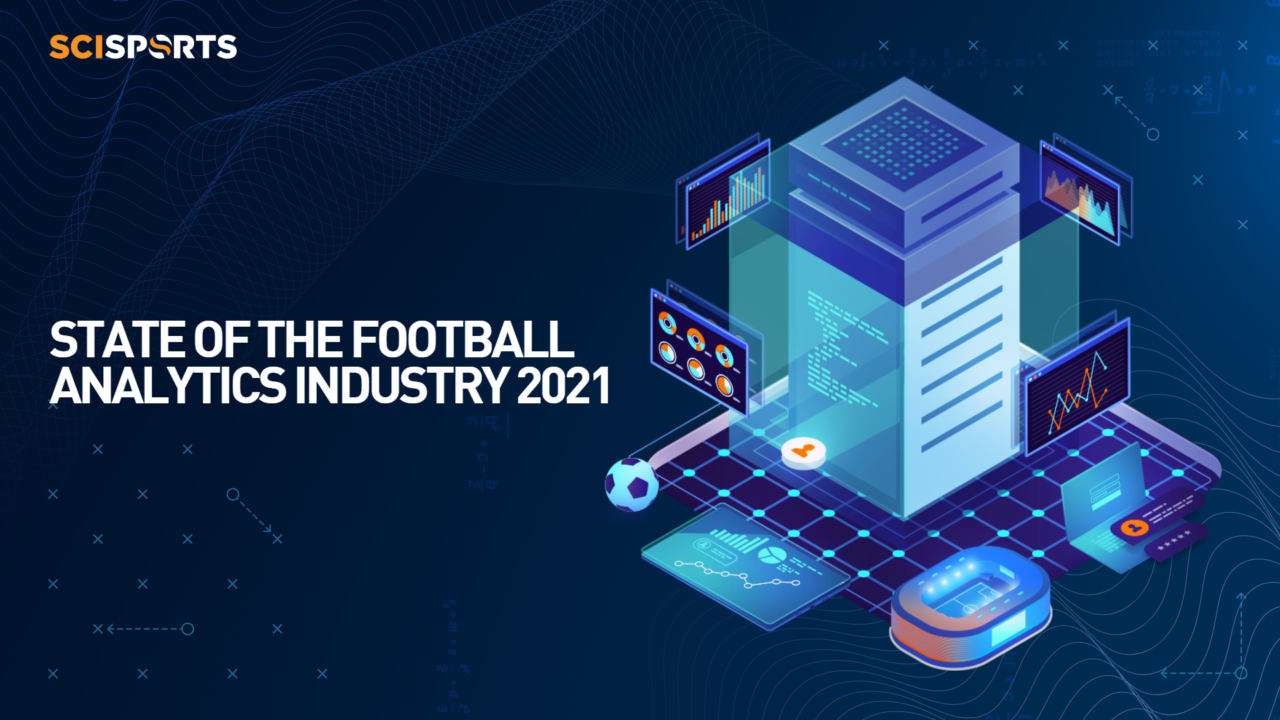
Technological advancements in football have seen a variety of data-related fields enter into the game. The introduction of data into professional football has been an innovative process and one that has included the emergence of different personas within the game.
Jan Van Haaren, Chief Analytics Officer at SciSports, has a unique position within the game. As the lead of data intelligence advancements within SciSports Labs, his role includes working on the very latest trends, developments and innovations in football analytics.
In this post, we speak to Jan Van Haaren about data intelligence in professional football, its development over time and much more.
Industry-leaders in football’s use of data intelligence
Just like competition on the field to be right at the very top, operations away from the pitch are equally competitive across several areas of football performance. The employment of data scientists and other personas of different non-footballing backgrounds has made the industry far more diverse.
As Van Haaren explains in depth here, certain strategies employed by clubs around the world have been done to varying effect over recent years.
“Many different types of football data exist and football clubs have adopted each type of data to a varying degree. The field is led by clubs with buy-in from the top to the bottom of the organisation, where the decision makers trust and know how to leverage insights from data,” Van Haaren noted.
“Most professional football clubs have used video data for opponent scouting and player recruitment as well as physical data collected via wearables to manage training load and to prevent injuries for many years already. Fewer football clubs are using match data collected via camera-based solutions and human annotators for opponent scouting and player recruitment, but the number of clubs that does so is steadily increasing.”
Beyond the implementation of data analytics into professional football clubs, Van Haaren points out how there are a number of ways that clubs can do this. From in-house teams to working externally with data intelligence companies, the possibilities are endless for professional clubs right now.
“The clubs that have bought into match data analysis can broadly be divided into two categories. On one hand, a select number of clubs have invested in in-house data analysis or data analytics departments. Clubs such as Midtjylland and Brentford have received widespread media attention for using data in their day-to-day operations. Clubs such as Liverpool, Barcelona, Manchester City and Leicester City have hired talented data scientists to carry out longer-term research projects… this list of clubs is likely still much longer, but many clubs prefer to remain secretive about their hires in this area hoping to gain a competitive advantage.
“On the other hand, an ever-increasing number of clubs is leveraging data by collaborating with specialised companies that offer data-driven tools for opposition scouting and player recruitment. For instance, SciSports offers an online platform that helps football clubs identify potential transfer targets in a matter of minutes with only a few mouse clicks. An increasing number of data-driven companies is offering competing products.
“Many football clubs somehow use data in their decision-making processes, but the way they do so and the extent to which they do so varies a lot from club to club. However, most clubs prefer to remain secretive about what they do in terms of data.”
How actionable insights help in professional football
While the increase in data accessibility and tooling has seen an increase in how frequently it is used within professional football, understanding how to use data points can be an equally valuable exercise.
“Many different types of football data exist these days and each type of data comes with its specific challenges. Video data and physical data like distance covered, number of high intensity sprints, number of acceleration have been around for quite some time and most clubs know how to deal with that kind of data.
“Match data tends to be more complex and larger in size and thus takes more skill and expertise to work with. For instance, optical tracking solutions that register the locations of the players and the ball at 25Hz generate a few million data points for each player per match. It is always better to have more match data though. Superfluous data can easily be omitted, whereas missing data is usually hard to collect.”
As Van Haaren points out, the availability of data is an extremely important element for football clubs. Yet once that data is collected and available to use, understanding the best way of comprehending what it actually says can be an entirely different challenge in itself.
“Football data poses many different challenges. One challenge is to collect and organise the data such that it can be analysed in an effective manner. The preparation of the data often takes more time than the actual analysis. Another challenge is to translate the questions from practitioners such as managers, coaches, sporting directors and scouts into formal problems that can be addressed with data and to translate the solutions to those problems back into answers in the football language that the practitioners use on a daily basis.
“Natural-language questions often involve a lot of uncertainty and vagueness which can be hard to translate to the data. For instance, when is the performance of a player perceived good or bad? What makes a potential transfer target a good fit or a bad fit from a tactical perspective? The answers to those questions should be formulated in such a way that practitioners understand them and can act upon them.”
Context is key for everyone involved
Following on from deriving actionable insights across an endless number of data points, analysts at clubs have a duty to provide the right context for coaches, players and other members of staff. Without the correct context for a statistic or data point, the right message is less likely to be relayed properly to improve on-field performances.
“Clubs should view data as an additional tool to make better-informed decisions. A better understanding of how your upcoming opponent will play or how a potential transfer target would perform at your club will contribute to achieving better results as a club.
“The key challenge for a club is to put the increasing amount of data that they have at their disposal in the right context and to draw the right conclusions from the data. However, the task of the analyst should be to present the data in such a way that the conclusions are obvious.”
The future of data usage in football
To conclude, Van Haaren identifies three key ways in which he sees data usage in football developing in future years. Across areas such as bridging the gap between analysts and coaches, increased predictive models and more, he believes that data usage in football has plenty of room to develop in the coming years.
“One direction is to better translate the data into answers to the questions that practitioners have in a language that they speak and understand. Practitioners are often presented with numbers that are hard to interpret because they are hard to relate to what happens on the pitch during a match. Data scientists and data analysts are still figuring out what is the most effective way to present insights from data to practitioners such as managers, coaches, sporting directors and scouts so that they can make the best possible decisions.
“Another direction is to transition from descriptive analytics to predictive and prescriptive analytics. Current data analysis techniques focus on analysing what has happened in the past, while most practitioners are much more interested in what will happen in the future or what decision would yield the most favourable outcome.
“Yet another direction is to apply advanced analytics to the development of individual players. Current data analysis mostly focuses on opposition scouting, player recruitment and post-match performance analysis. However, the available data could also be used to identify and develop the weaknesses of players who are already on their team. For instance, if the data suggests that a given player is poor at making the right decision in the final third of the pitch, then a tailored training program could be devised to help the player improve their decision-making skills in those situations.”
RECRUITMENT
Find the players you're looking for
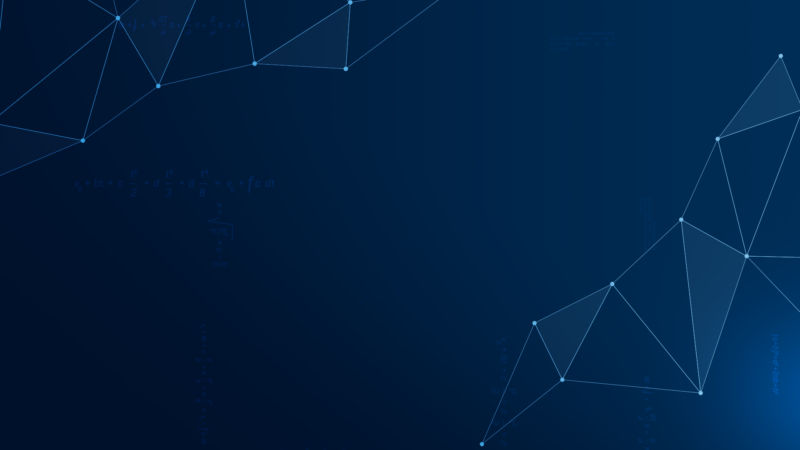
PERFORMANCE ANALYSIS
The smartest physical and tactical data insights
